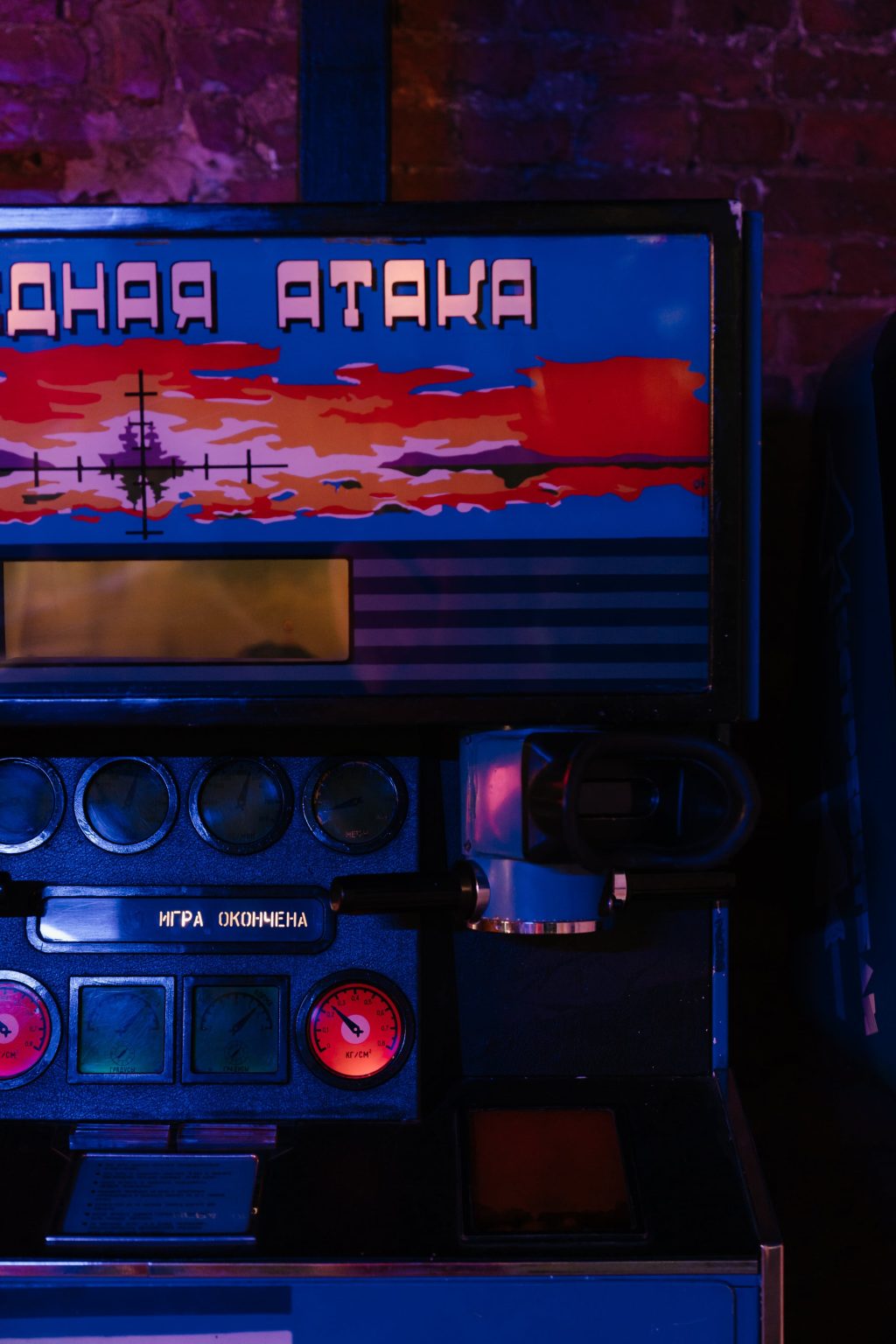
With the rapid advancements in technology, video games have become increasingly sophisticated, captivating players across the globe. However, a major challenge faced by game developers is finding the right balance between providing a thrilling and engaging experience while ensuring that players aren’t overwhelmed or bored by the difficulty level. This is where machine learning has emerged as a vital tool in dynamic game difficulty adjustment.
Understanding Dynamic Game Difficulty Adjustment
Dynamic game difficulty adjustment (DGDA) refers to the ability of a game to adapt its difficulty level in real-time, based on the player’s actions, skill level, and other relevant factors. This adjustment ensures that players are continuously challenged, promoting enhanced immersion and satisfaction. Gone are the days of fixed difficulty levels that frustrate players or bore them after mastering a particular skill.
The Benefits of Machine Learning
Machine learning, a subset of artificial intelligence, plays a crucial role in empowering games with adaptive difficulty levels. By analyzing vast amounts of player data, machine learning algorithms can dynamically adjust various elements of gameplay, such as enemy AI behavior, spawn rates, power-up distribution, puzzles, and more. This results in a personalized gaming experience that is tailored to each player’s unique skill set.
Player Profiling
One of the fundamental aspects of machine learning in DGDA is player profiling. By collecting and analyzing data related to player performance, behavior, and preferences, the algorithm builds a detailed profile for each player. This profile becomes the foundation for adjusting the gameplay elements to provide the ideal level of challenge and engagement.
Adaptive AI
Machine learning algorithms can create adaptive artificial intelligence (AI) systems that learn from player behavior. These AI entities continuously assess the player’s performance, adapting and evolving their strategies to pose an appropriate level of challenge. This ensures that players face enemies who intelligently respond to their actions, promoting a sense of accomplishment while keeping the gameplay engaging.
Content Generation
DGDA powered by machine learning algorithms can also streamline content generation. By analyzing player behavior and preferences, the algorithm can dynamically generate new challenges, puzzles, levels, and missions. This ability to create personalized content not only keeps players engaged but also increases the replayability factor, allowing them to discover new experiences with each playthrough.
Real-Time Feedback
Machine learning enables real-time feedback mechanisms that provide players with hints, tips, or slightly adjusted gameplay to ensure they are neither overwhelmed nor bored. The algorithm can detect when a player is struggling and intervene, offering assistance to help them progress without ruining the sense of accomplishment. On the other hand, if a player is finding the game too easy, the system can introduce new challenges to maintain engagement.
Conclusion
As the gaming industry continues to evolve, finding the perfect balance between challenge and fun becomes increasingly crucial. Machine learning has significantly transformed the development of dynamic difficulty adjustment in games. By leveraging player profiling, adaptive AI, content generation, and real-time feedback, game developers can deliver personalized experiences that cater to each player’s skills, preferences, and enjoyment. The integration of machine learning in DGDA not only enhances player satisfaction but also sets the stage for even more immersive and captivating gaming experiences in the future.